In simple terms, “edge” or “fog” computing refers to processing things closer to their source (i.e., at the edge of a network) vs. large centralized systems that serve all data requests from a single resource.
The market for Edge AI hardware is anticipated to increase from 1,056 million units in 2022 to 2,716 million units by 2027, growing at a CAGR of 20.8% over the forecast period.
Quite a few people are discussing it as the next big thing in cloud computing. While there’s no specific infrastructure or standard here to apply, edge computing should be considered for deploying applications and services. In this article, we’ll look at real-world use cases of edge computing.
An Overview of Edge Computing
Edge computing is a way of processing data closer to where it is generated. It shifts data processing outside the cloud and onto devices where a local machine can analyze it.
According to the research, the global edge computing market will reach a value of USD 116.5 billion by 2030, growing at a CAGR of 12.46% from 2022 to 2030. Edge computing can potentially bring several benefits for both businesses and consumers. These include faster response times, reduced latency, increased privacy, and improved security.
The concept of “edge computing” isn’t new; companies have used edge servers for years to process data before sending it back to their main data centers for analysis. However, there are several reasons why this has become more popular recently:
- Rising Demand for Real-time Processing: With the proliferation of IoT and AI applications, the need for instant processing and response times has surged.
- Latency-sensitive Applications: Industries like autonomous vehicles, robotics, and industrial automation require minimal latency for safe and efficient operations.
- Bandwidth Optimization: Reducing the load on central cloud servers by processing data locally helps optimize network resources.
- Data Privacy and Security Concerns: Edge AI mitigates data privacy risks by processing sensitive information on-device, minimizing exposure to external networks.
- Unreliable Connectivity: Edge AI enables devices to function without consistent internet access, which is crucial for remote or harsh environments.
- Energy Efficiency: Local processing on edge devices consumes less power than transmitting data back and forth to distant cloud servers.
- Cost-effectiveness: Edge AI reduces the need for continuous high-bandwidth communication, leading to cost savings.
- Regulatory Compliance: In certain industries, local processing helps adhere to data residency and compliance regulations more effectively.
Advantages of Edge Computing
The main goal is to reduce latency and bandwidth usage by bringing the data processing and storage closer to the users.
The advantages of edge computing are:
- Reduced Latency: The latency is the time it takes for a packet to travel from its source to its destination. When data is processed at the edge, less time is needed to process that data, resulting in faster response times.
- Faster Response Times: Faster response times mean businesses can deliver services more quickly and efficiently than ever. As a result, customers will get the information they want faster and be able to make decisions based on real-time data. This can make all the difference in business success in today’s marketplace.
- Enhanced Security: By moving processing closer to where data is generated, organizations can significantly improve their network security by reducing the risk of cyberattacks and other security threats that could compromise sensitive data stored in central locations like servers or databases.
- Improved Privacy: Since all the data processing happens on your device, it gives you more control over your personal information and data. You can easily configure your device to protect your sensitive information from hackers and other online threats like viruses or malware attacks.
Use Cases Examples of Edge Computing
The need for data processing at the edge is growing as the world becomes increasingly connected. The edge is where data is generated, and decisions are made. It’s also where much computing power is needed to run complex algorithms. As a result, many companies are turning to edge computing to solve their problems. Here are some examples:
Autonomous Vehicles
Autonomous vehicles are a great example of edge computing. Autonomous vehicles need to be able to make real-time decisions and have very limited bandwidth.
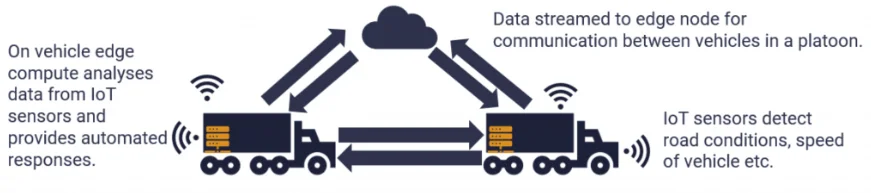
Edge computing allows autonomous vehicles to make decisions based on the data coming in from sensors on the car and other connected devices nearby.
The main benefit is that autonomous vehicles can make much better decisions about what is happening around them because they can access more information than a centralized system would allow. Autonomous vehicles can also share information before it gets sent back to the cloud.
This means that if one vehicle sees something happening on the road ahead, it can send that information directly to all other cars around it so they know how to react.
Face Recognition System
Face recognition is a computer-based technology for identifying a person from a digital image or a video frame from a video source. It is also known as face identification, although the latter may be confused with other technologies such as facial recognition and biometric identification.
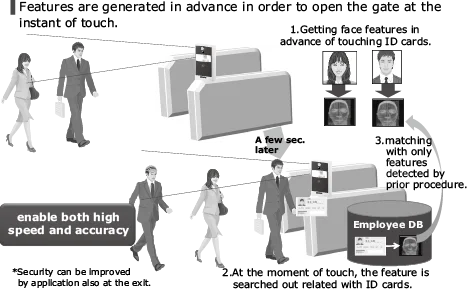
Face recognition has many applications in security systems and law enforcement. For example, it is used to identify bank robbery suspects by comparing surveillance photos with mug shots. Another application can be used to identify people in casinos who are banned from playing certain games because they are thought to be cheaters.
A system like this can also determine if an individual buys alcohol while underage based on a database of photos of people under 21.
Real-Time Food Security & Safety Monitoring
In the food industry, temperature monitoring is critical because it can help prevent food spoilage, which can lead to illness. With the right technology, it’s possible to monitor temperatures in various parts of a facility and send alerts if any areas deviate from expected values. This allows you to take action before your product becomes unsafe for consumption.
Microsoft Azure IoT Edge
Microsoft Azure IoT Edge is a fully managed service that provides a self-contained, secure, and hardened runtime environment for your applications. It supports the execution of lightweight machine learning algorithms on the edge device and enables you to run your application code in a secure containerized environment.
With Azure IoT Edge, you can run your code in a container directly on the IoT device or any cloud. Microsoft Azure IoT Edge also supports an event-driven programming model.
Intelligent Transportation Systems (ITS)
In the transportation sector, ITS involves using vehicle sensors to collect data about traffic conditions. This information can then be used to improve traffic flow and reduce congestion. The data generated from these sensors can be processed at the edge device or in the cloud. However, when dealing with large amounts of data, it is often better to process it locally as this will increase processing speeds.
Smart Surveillance
In smart surveillance, capturing and analyzing large amounts of data in real-time is important. Edge computing can be used to improve the speed at which a video camera can detect a person or object.
The camera will send images to the edge cloud for processing, where they can be analyzed in real-time, and alerts sent back immediately. This is a critical feature for law enforcement, security, and retail store monitoring applications.
Kiosk Machines
Edge computing can provide real-time analytics on kiosk machines (e.g., vending machines). This can help improve product availability, reduce theft, and increase sales performance. For example, if no beverages are available at a vending machine, the vendor would receive an alert immediately to dispatch someone to refill the machine with products.
A similar use case could be applied to supermarket self-checkout counters where customers need immediate service when no items are available for sale or checkout procedures aren’t working properly due to system malfunctions or human error.
Kitchen Temperature/Humidity Monitoring for Food Security and Safety
In the food industry, food safety is a top priority. For example, restaurants must ensure that their kitchens are always kept at the correct temperature.
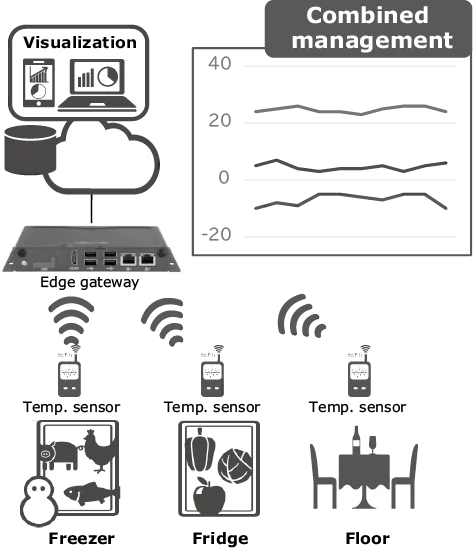
If a restaurant’s kitchen gets too hot or cold, this can impact the quality of its products and even lead to health issues for consumers. The same applies to other environments, such as warehouses and cold storage facilities. In this case, edge computing can monitor temperature and humidity levels with real-time data collection.
This data is then sent back to a centralized hub to be analyzed and used to alert staff if there are any environmental problems. This can help companies ensure that their products remain safe for consumption by customers.